Open Access
ARTICLE
A Step-Based Deep Learning Approach for Network Intrusion Detection
1 Jilin Business and Technology College, Changchun, 130507, China
2 College of Earth Sciences, Jilin University, Changchun, 130061, China
* Corresponding Author: Xiangjin Ran. Email:
(This article belongs to the Special Issue: Swarm Intelligence and Applications in Combinatorial Optimization)
Computer Modeling in Engineering & Sciences 2021, 128(3), 1231-1245. https://doi.org/10.32604/cmes.2021.016866
Received 04 April 2021; Accepted 27 May 2021; Issue published 11 August 2021
Abstract
In the network security field, the network intrusion detection system (NIDS) is considered one of the critical issues in the detection accuracy and missed detection rate. In this paper, a method of two-step network intrusion detection on the basis of GoogLeNet Inception and deep convolutional neural networks (CNNs) models is proposed. The proposed method used the GoogLeNet Inception model to identify the network packets’ binary problem. Subsequently, the characteristics of the packets’ raw data and the traffic features are extracted. The CNNs model is also used to identify the multiclass intrusions by the network packets’ features. In the experimental results, the proposed method shows an improvement in the identification accuracy, where it achieves up to 99.63%. In addition, the missed detection rate is reduced to be 0.1%. The results prove the high performance of the proposed method in enhancing the NIDS’s reliability.Keywords
Cite This Article
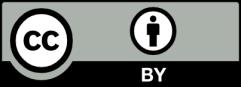
This work is licensed under a Creative Commons Attribution 4.0 International License , which permits unrestricted use, distribution, and reproduction in any medium, provided the original work is properly cited.