Open Access
ARTICLE
Global and Graph Encoded Local Discriminative Region Representation for Scene Recognition
1 School of Medical Instrument and Food Engineering, University of Shanghai for Science and Technology, Shanghai, 200093, China
2 Major of Electrical Engineering and Electronics, Graduate School of Engineering, Kogakuin University, Tokyo, 163-8677, Japan
* Corresponding Authors: Feifei Lee. Email: ; Qiu Chen. Email:
# Both authors contributed equally to this work
Computer Modeling in Engineering & Sciences 2021, 128(3), 985-1006. https://doi.org/10.32604/cmes.2021.014522
Received 05 October 2020; Accepted 19 May 2021; Issue published 11 August 2021
Abstract
Scene recognition is a fundamental task in computer vision, which generally includes three vital stages, namely feature extraction, feature transformation and classification. Early research mainly focuses on feature extraction, but with the rise of Convolutional Neural Networks (CNNs), more and more feature transformation methods are proposed based on CNN features. In this work, a novel feature transformation algorithm called Graph Encoded Local Discriminative Region Representation (GEDRR) is proposed to find discriminative local representations for scene images and explore the relationship between the discriminative regions. In addition, we propose a method using the multi-head attention module to enhance and fuse convolutional feature maps. Combining the two methods and the global representation, a scene recognition framework called Global and Graph Encoded Local Discriminative Region Representation (G2ELDR2) is proposed. The experimental results on three scene datasets demonstrate the effectiveness of our model, which outperforms many state-of-the-arts.Keywords
Cite This Article
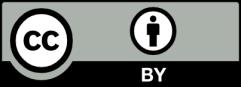