Open Access
ARTICLE
Intelligent Segmentation and Measurement Model for Asphalt Road Cracks Based on Modified Mask R-CNN Algorithm
1 School of Water Conservancy Engineering, Zhengzhou University, Zhengzhou, 450001, China
2 National Local Joint Engineering Laboratory of Major Infrastructure Testing and Rehabilitation Technology, Zhengzhou, 450001, China
3 Collaborative Innovation Center of Water Conservancy and Transportation Infrastructure Safety, Zhengzhou, 450001, China
4 Guangzhou Expressway Limited Company, Guangzhou, 510288, China
* Corresponding Author: Niannian Wang. Email:
Computer Modeling in Engineering & Sciences 2021, 128(2), 541-564. https://doi.org/10.32604/cmes.2021.015875
Received 20 January 2021; Accepted 19 April 2021; Issue published 22 July 2021
Abstract
Nowadays, asphalt road has dominated highways around the world. Among various defects of asphalt road, cracks have been paid more attention, since cracks often cause major engineering and personnel safety incidents. Current manual crack inspection methods are time-consuming and labor-intensive, and most segmentation methods cannot detect cracks at the pixel level. This paper proposes an intelligent segmentation and measurement model based on the modified Mask R-CNN algorithm to automatically and accurately detect asphalt road cracks. The model proposed in this paper mainly includes a convolutional neural network (CNN), an optimized region proposal network (RPN), a region of interest (RoI) Align layer, a candidate area classification network and a Mask branch of fully convolutional network (FCN). The ratio and size of anchors in the RPN are adjusted to improve the accuracy and efficiency of segmentation. Soft non-maximum suppression (Soft-NMS) algorithm is developed to improve the segmentation accuracy. A dataset including 8,689 images (512 × 512 pixels) of asphalt cracks is established and the road crack is manually marked. Transfer learning is used to initialize the model parameters in the training process. To optimize the model training parameters, multiple comparison experiments are performed, and the test results show that the mean average precision (mAP) value and F1-score of the optimal trained model are 0.952 and 0.949. Subsequently, the robustness verification test and comparative test of the trained model are conducted and the topological features of the crack are extracted. Then, the damage area, length and average width of the crack are measured automatically and accurately at pixel level. More importantly, this paper develops an automatic crack detection platform for asphalt roads to automatically extract the number, area, length and average width of cracks, which can significantly improve the crack detection efficiency for the road maintenance industry.Keywords
Cite This Article
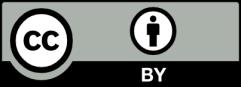
This work is licensed under a Creative Commons Attribution 4.0 International License , which permits unrestricted use, distribution, and reproduction in any medium, provided the original work is properly cited.