Open Access
ARTICLE
Deep Learning-Based Surrogate Model for Flight Load Analysis
1 College of Aerospace Science and Engineering, National University of Defense Technology, Changsha, 410073, China
2 School of Science, Harbin Institute of Technology, Shenzhen, 518000, China
3 National Innovation Institute of Defense Technology, Chinese Academy of Military Science, Beijing, 100071, China
* Corresponding Author: Qinghui Zhang. Email:
Computer Modeling in Engineering & Sciences 2021, 128(2), 605-621. https://doi.org/10.32604/cmes.2021.015747
Received 10 January 2021; Accepted 26 April 2021; Issue published 22 July 2021
Abstract
Flight load computations (FLC) are generally expensive and time-consuming. This paper studies deep learning (DL)-based surrogate models of FLC to provide a reliable basis for the strength design of aircraft structures. We mainly analyze the influence of Mach number, overload, angle of attack, elevator deflection, altitude, and other factors on the loads of key monitoring components, based on which input and output variables are set. The data used to train and validate the DL surrogate models are derived using aircraft flight load simulation results based on wind tunnel test data. According to the FLC features, a deep neural network (DNN) and a random forest (RF) are proposed to establish the surrogate models. The DNN meets the FLC accuracy requirement using rich data sources in the FLC; the RF can alleviate overfitting and evaluate the importance of flight parameters. Numerical experiments show that both the DNN-and RF-based surrogate models achieve high accuracy. The input variables importance analysis demonstrates that vertical overload and elevator deflection have a significant influence on the FLC. We believe that synthetic applications of these DL-based surrogate methods show a great promise in the field of FLC.Keywords
Cite This Article
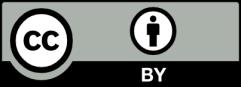