Open Access
ARTICLE
An Improved Algorithm for the Detection of Fastening Targets Based on Machine Vision
College of Nuclear Technology and Automation Engineering, Sichuan Key Laboratory of Geoscience Nuclear Technology, Chengdu University of Technology, Chengdu, 610059, China
* Corresponding Author: Haihui Huang. Email:
# These authors contributed equally to this work.
(This article belongs to the Special Issue: Machine Learning based Methods for Mechanics)
Computer Modeling in Engineering & Sciences 2021, 128(2), 779-802. https://doi.org/10.32604/cmes.2021.014993
Received 14 November 2020; Accepted 31 March 2021; Issue published 22 July 2021
Abstract
Object detection plays an important role in the sorting process of mechanical fasteners. Although object detection has been studied for many years, it has always been an industrial problem. Edge-based model matching is only suitable for a small range of illumination changes, and the matching accuracy is low. The optical flow method and the difference method are sensitive to noise and light, and camshift tracking is less effective in complex backgrounds. In this paper, an improved target detection method based on YOLOv3-tiny is proposed. The redundant regression box generated by the prediction network is filtered by soft nonmaximum suppression (NMS) instead of the hard decision NMS algorithm. This not only increases the size of the network structure by 52 × 52 and improves the detection accuracy of small targets but also uses the basic structure block MobileNetv2 in the feature extraction network, which enhances the feature extraction ability with the increased network layer and improves network performance. The experimental results show that the improved YOLOv3-tiny target detection algorithm improves the detection ability of bolts, nuts, screws and gaskets. The accuracy of a single type has been improved, which shows that the network greatly enhances the ability to learn objects with slightly complex features. The detection result of single shape features is slightly improved, which is higher than the recognition accuracy of other types. The average accuracy is increased from 0.813 to 0.839, an increase of two percentage points. The recall rate is increased from 0.804 to 0.821.Keywords
Cite This Article
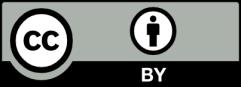
This work is licensed under a Creative Commons Attribution 4.0 International License , which permits unrestricted use, distribution, and reproduction in any medium, provided the original work is properly cited.