Open Access
ARTICLE
MRI Brain Tumor Segmentation Using 3D U-Net with Dense Encoder Blocks and Residual Decoder Blocks
1 College of Computer Science, Sichuan University, Chengdu, 610065, China
2 School of Software Engineering, Chengdu University of Information Technology, Chengdu, 610225, China
3 School of Computer Science, Chengdu University of Information Technology, Chengdu, 610225, China
* Corresponding Authors: Juhong Tie. Email: ; Jiliu Zhou. Email:
(This article belongs to the Special Issue: Recent Advances on Deep Learning for Medical Signal Analysis (RADLMSA))
Computer Modeling in Engineering & Sciences 2021, 128(2), 427-445. https://doi.org/10.32604/cmes.2021.014107
Received 01 September 2020; Accepted 12 May 2021; Issue published 22 July 2021
Abstract
The main task of magnetic resonance imaging (MRI) automatic brain tumor segmentation is to automatically segment the brain tumor edema, peritumoral edema, endoscopic core, enhancing tumor core and nonenhancing tumor core from 3D MR images. Because the location, size, shape and intensity of brain tumors vary greatly, it is very difficult to segment these brain tumor regions automatically. In this paper, by combining the advantages of DenseNet and ResNet, we proposed a new 3D U-Net with dense encoder blocks and residual decoder blocks. We used dense blocks in the encoder part and residual blocks in the decoder part. The number of output feature maps increases with the network layers in contracting path of encoder, which is consistent with the characteristics of dense blocks. Using dense blocks can decrease the number of network parameters, deepen network layers, strengthen feature propagation, alleviate vanishing-gradient and enlarge receptive fields. The residual blocks were used in the decoder to replace the convolution neural block of original U-Net, which made the network performance better. Our proposed approach was trained and validated on the BraTS2019 training and validation data set. We obtained dice scores of 0.901, 0.815 and 0.766 for whole tumor, tumor core and enhancing tumor core respectively on the BraTS2019 validation data set. Our method has the better performance than the original 3D U-Net. The results of our experiment demonstrate that compared with some state-of-the-art methods, our approach is a competitive automatic brain tumor segmentation method.Keywords
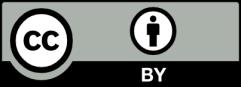