Open Access
ARTICLE
A Mortality Risk Assessment Approach on ICU Patients Clinical Medication Events Using Deep Learning
1 School of Computer and Information Engineering, Hunan University of Technology and Business, Changsha, 410205, China
2 Department of Computer Science, The University of Pittsburgh, Pittsburgh, PA 15213, USA
* Corresponding Author: Hanzhong Zheng. Email:
(This article belongs to the Special Issue: Recent Advances on Deep Learning for Medical Signal Analysis (RADLMSA))
Computer Modeling in Engineering & Sciences 2021, 128(1), 161-181. https://doi.org/10.32604/cmes.2021.014917
Received 07 November 2020; Accepted 30 March 2021; Issue published 28 June 2021
Abstract
ICU patients are vulnerable to medications, especially infusion medications, and the rate and dosage of infusion drugs may worsen the condition. The mortality prediction model can monitor the real-time response of patients to drug treatment, evaluate doctors’ treatment plans to avoid severe situations such as inverse Drug-Drug Interactions (DDI), and facilitate the timely intervention and adjustment of doctor’s treatment plan. The treatment process of patients usually has a time-sequence relation (which usually has the missing data problem) in patients’ treatment history. The state-of-the-art method to model such time-sequence is to use Recurrent Neural Network (RNN). However, sometimes, patients’ treatment can last for a long period of time, which RNN may not fit for modelling long time sequence data. Therefore, we propose to use the heterogeneous medication events driven LSTM to predict the outcome of the patient, and the Natural Language Processing and Gaussian Process (GP), which can handle noisy, incomplete, sparse, heterogeneous and unevenly sampled patients’ medication records. In our work, we emphasize the semantic meaning of each medication event and the sequence of the medication events on patients, while also handling the missing value problem using kernel-based Gaussian process. We compare the performance of LSTM and Phased-LSTM on modelling the outcome of patients’ treatment and data imputation using kernel-based Gaussian process and conduct an empirical study on different data imputation approaches.Keywords
Cite This Article
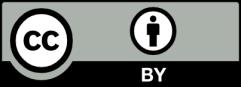