Open Access
ARTICLE
Classification of Domestic Refuse in Medical Institutions Based on Transfer Learning and Convolutional Neural Network
1
School of Control Engineering, Chengdu University of Information Technology, Chengdu, 610225, China
2
College of Automation, Chongqing University of Posts and Telecommunications, Chongqing, 400065, China
3
Department of Informatics, University of Leicester, Leicester, LE1 7RH, UK
* Corresponding Author: Hanbing Yan. Email:
(This article belongs to the Special Issue: Recent Advances on Deep Learning for Medical Signal Analysis (RADLMSA))
Computer Modeling in Engineering & Sciences 2021, 127(2), 599-620. https://doi.org/10.32604/cmes.2021.014119
Received 01 September 2020; Accepted 01 February 2021; Issue published 19 April 2021
Abstract
The problem of domestic refuse is becoming more and more serious with the use of all kinds of equipment in medical institutions. This matter arouses people’s attention. Traditional artificial waste classification is subjective and cannot be put accurately; moreover, the working environment of sorting is poor and the efficiency is low. Therefore, automated and effective sorting is needed. In view of the current development of deep learning, it can provide a good auxiliary role for classification and realize automatic classification. In this paper, the ResNet-50 convolutional neural network based on the transfer learning method is applied to design the image classifier to obtain the domestic refuse classification with high accuracy. By comparing the method designed in this paper with back propagation neural network and convolutional neural network , it is concluded that the CNN based on transfer learning method applied in this paper with higher accuracy rate and lower false detection rate. Further, under the shortage situation of data samples, the method with transfer learning and ResNet-50 training model is effective to improve the accuracy of image classification.Keywords
Cite This Article
Citations
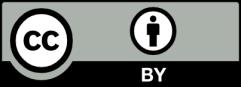
This work is licensed under a Creative Commons Attribution 4.0 International License , which permits unrestricted use, distribution, and reproduction in any medium, provided the original work is properly cited.