Open Access
ARTICLE
Traffic Anomaly Detection Method Based on Improved GRU and EFMS-Kmeans Clustering
1 Science and Technology on Communication Networks Laboratory, The 54th Research Institute of CETC, Shijiazhuang, China
2 Department of Military Representative Office of General Military Equipment Development Shijiazhuang, Shijiazhuang, China
3 State Key Laboratory of Networking and Switching Technology, Beijing University of Posts and Telecommunications, Beijing, 100876, China
* Corresponding Author: Yang Yang. Email:
Computer Modeling in Engineering & Sciences 2021, 126(3), 1053-1091. https://doi.org/10.32604/cmes.2021.013045
Received 23 July 2020; Accepted 04 November 2020; Issue published 19 February 2021
Abstract
In recent years, with the continuous development of information technology and the rapid growth of network scale, network monitoring and management become more and more important. Network traffic is an important part of network state. In order to ensure the normal operation of the network, improve the availability of the network, find network faults in time and deal with network attacks; it is necessary to detect the abnormal traffic in the network. Abnormal traffic detection is of great significance in the actual network management. Therefore, in order to improve the accuracy and efficiency of network traffic anomaly detection, this paper proposes a comprehensive anomaly detection method based on improved GRU traffic prediction and improved K-means clustering, and cascade the traffic prediction and clustering to achieve the purpose of anomaly detection. Firstly, an improved highway-GRU algorithm HS-GRU (An improved Gate Recurrent Unit neural network based on Highway network and STL algorithm, HS-GRU) is proposed, which combines STL decomposition algorithm with highway GRU neural network and uses this improved algorithm to predict traffic. And then, we proposed the EFMS-Kmeans algorithm (An improved clustering algorithm that combined Mean Shift algorithm based on electrostatic force with K-means clustering) to solve the shortcoming of the traditional K-means clustering which cannot automatically determine the number of clustering. The sum of the squared errors (SSE) method and the contour coefficient method were used to double test the clustering effect. After determining the clustering center, the potential energy gradient was directly used for anomaly detection by using the threshold method, which considered the local characteristics of the data and ensured the accuracy of anomaly detection. The simulation results show that the anomaly detection algorithm based on HS-GRU and EFMS-Kmeans clustering proposed in this paper can effectively improve the accuracy of flow anomaly detection and has important application value.Keywords
Cite This Article
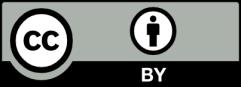