Open Access
ARTICLE
Prediction of Permeability Using Random Forest and Genetic Algorithm Model
1 Key Laboratory of Deep Coal Resource Mining (CUMT), Ministry of Education of China, School of Mines, China University of Mining and Technology, Xuzhou, 221116, China
2 College of Safety and Emergency Management Engineering, Taiyuan University of Technology, Taiyuan 030024, China
3 China Energy Investment Group Company, Beijing, 100011, China
∗ Corresponding Authors: Zhijun Wan. Email: ; Yi Wang. Email:
(This article belongs to the Special Issue: Modeling and Simulation of Fluid flows in Fractured Porous Media: Current Trends and Prospects)
Computer Modeling in Engineering & Sciences 2020, 125(3), 1135-1157. https://doi.org/10.32604/cmes.2020.014313
Received 17 September 2020; Accepted 26 October 2020; Issue published 15 December 2020
Abstract
Precise recovery of Coalbed Methane (CBM) based on transparent reconstruction of geological conditions is a branch of intelligent mining. The process of permeability reconstruction, ranging from data perception to real-time data visualization, is applicable to disaster risk warning and intelligent decision-making on gas drainage. In this study, a machine learning method integrating the Random Forest (RF) and the Genetic Algorithm (GA) was established for permeability prediction in the Xishan Coalfield based on Uniaxial Compressive Strength (UCS), effective stress, temperature and gas pressure. A total of 50 sets of data collected by a self-developed apparatus were used to generate datasets for training and validating models. Statistical measures including the coefficient of determination (R2) and Root Mean Square Error (RMSE) were selected to validate and compare the predictive performances of the single RF model and the hybrid RF– GA model. Furthermore, sensitivity studies were conducted to evaluate the importance of input parameters. The results show that, the proposed RF–GA model is robust in predicting the permeability; UCS is directly correlated to permeability, while all other inputs are inversely related to permeability; the effective stress exerts the greatest impact on permeability based on importance score, followed by the temperature (or gas pressure) and UCS. The partial dependence plots, indicative of marginal utility of each feature in permeability prediction, are in line with experimental results. Thus, the proposed hybrid model (RF–GA) is capable of predicting permeability and thus beneficial to precise CBM recovery.Keywords
Cite This Article
Citations
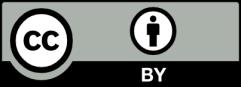