Open Access
ARTICLE
PDNet: A Convolutional Neural Network Has Potential to be Deployed on Small Intelligent Devices for Arrhythmia Diagnosis
1 Department of Computer Science and Engineering, Southern University of Science and Technology, Shenzhen, China
2 Cooperative Innovtion Center of Internet Healthcare, Zhengzhou University, Zhengzhou, 450052, China
3 College of Computer Science and Technology, Huaqiao University, Xiamen, 361021, China
* Corresponding Author: Xiaoqing Zhang. Email:
# Fei Yang and Xiaoqing Zhang contributed equally to this work
(This article belongs to the Special Issue: Recent Advances on Deep Learning for Medical Signal Analysis (RADLMSA))
Computer Modeling in Engineering & Sciences 2020, 125(1), 365-382. https://doi.org/10.32604/cmes.2020.010798
Received 21 March 2020; Accepted 20 July 2020; Issue published 18 September 2020
Abstract
Heart arrhythmia is a group of irregular heartbeat conditions and is usually detected by electrocardiograms (ECG) signals. Over the past years, deep learning methods have been developed to classify different types of heart arrhythmias through ECG based on computer-aided diagnosis systems (CADs), but these deep learning methods usually cannot trade-off between classification performance and parameters of deep learning methods. To tackle this problem, this work proposes a convolutional neural network (CNN) model named PDNet to recognize different types of heart arrhythmias efficiently. In the PDNet, a convolutional block named PDblock is devised, which is comprised of a pointwise convolutional layer and a depthwise convolutional layer. Furthermore, an improved loss function is utilized to improve the results of heart arrhythmias classification. To verify the proposed CNN model, extensive experiments are conducted on public MIT-BIH ECG databases. The experimental results demonstrate that the proposed PDNet achieves an accuracy of 98.2% accuracy and outperforms state-of-the-art methods about 2%.Keywords
Cite This Article
Citations
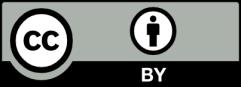
This work is licensed under a Creative Commons Attribution 4.0 International License , which permits unrestricted use, distribution, and reproduction in any medium, provided the original work is properly cited.