Open Access
ARTICLE
A Multi-View Gait Recognition Method Using Deep Convolutional Neural Network and Channel Attention Mechanism
College of Engineering, Huaqiao University, Quanzhou, 362021, China
* Corresponding Author: Jiabin Wang. Email:
(This article belongs to the Special Issue: Recent Advances on Deep Learning for Medical Signal Analysis (RADLMSA))
Computer Modeling in Engineering & Sciences 2020, 125(1), 345-363. https://doi.org/10.32604/cmes.2020.011046
Received 16 April 2020; Accepted 20 July 2020; Issue published 18 September 2020
Abstract
In many existing multi-view gait recognition methods based on images or video sequences, gait sequences are usually used to superimpose and synthesize images and construct energy-like template. However, information may be lost during the process of compositing image and capture EMG signals. Errors and the recognition accuracy may be introduced and affected respectively by some factors such as period detection. To better solve the problems, a multi-view gait recognition method using deep convolutional neural network and channel attention mechanism is proposed. Firstly, the sliding time window method is used to capture EMG signals. Then, the back-propagation learning algorithm is used to train each layer of convolution, which improves the learning ability of the convolutional neural network. Finally, the channel attention mechanism is integrated into the neural network, which will improve the ability of expressing gait features. And a classifier is used to classify gait. As can be shown from experimental results on two public datasets, OULP and CASIA-B, the recognition rate of the proposed method can be achieved at 88.44% and 97.25% respectively. As can be shown from the comparative experimental results, the proposed method has better recognition effect than several other newer convolutional neural network methods. Therefore, the combination of convolutional neural network and channel attention mechanism is of great value for gait recognition.Keywords
Cite This Article
Citations
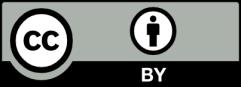
This work is licensed under a Creative Commons Attribution 4.0 International License , which permits unrestricted use, distribution, and reproduction in any medium, provided the original work is properly cited.