Open Access
ARTICLE
Least-Square Support Vector Machine and Wavelet Selection for Hearing Loss Identification
1 School of Computer Science and Technology, Henan Polytechnic University, Jiaozuo, 454000, China
2 Department of Computer Science and Engineering, Malaviya National Institute of Technology, Jaipur, 302017, India
3 School of Mathematics and Actuarial Science, University of Leicester, Leicester, LE1 7RH, UK
4 Department of Information Systems, Faculty of Computing and Information Technology, King Abdulaziz University, Jeddah, 21589, Saudi Arabia
* Corresponding Author: Shuihua Wang. Email:
(This article belongs to the Special Issue: Recent Advances on Deep Learning for Medical Signal Analysis (RADLMSA))
Computer Modeling in Engineering & Sciences 2020, 125(1), 299-313. https://doi.org/10.32604/cmes.2020.011069
Received 17 April 2020; Accepted 30 June 2020; Issue published 18 September 2020
Abstract
Hearing loss (HL) is a kind of common illness, which can significantly reduce the quality of life. For example, HL often results in mishearing, misunderstanding, and communication problems. Therefore, it is necessary to provide early diagnosis and timely treatment for HL. This study investigated the advantages and disadvantages of three classical machine learning methods: multilayer perceptron (MLP), support vector machine (SVM), and least-square support vector machine (LS-SVM) approach and made a further optimization of the LS-SVM model via wavelet entropy. The investigation illustrated that themultilayer perceptron is a shallowneural network,while the least square support vector machine uses hinge loss function and least-square optimization method. Besides, a wavelet selection method was proposed, and we found db4 can achieve the best results. The experiments showed that the LS-SVM method can identify the hearing loss disease with an overall accuracy of three classes as 84.89 ± 1.77, which is superior to SVM and MLP. The results show that the least-square support vector machine is effective in hearing loss identification.Keywords
Cite This Article
Citations
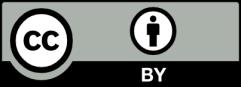
This work is licensed under a Creative Commons Attribution 4.0 International License , which permits unrestricted use, distribution, and reproduction in any medium, provided the original work is properly cited.