Open Access
ARTICLE
Threshold-Based Adaptive Gaussian Mixture Model Integration (TA-GMMI) Algorithm for Mapping Snow Cover in Mountainous Terrain
1 School of Automation, Nanjing University of Information Science & Technology, Nanjing, 210044, China
2 Jiangsu Collaborative Innovation Center of Atmospheric Environment and Equipment Technology, Nanjing University of Information Science & Technology, Nanjing, 210044, China
3 School of Computer and Software, Nanjing University of Information Science & Technology, Nanjing, 210044, China
4 School of Atmospheric Physics, Nanjing University of Information Science & Technology, Nanjing, 210044, China
* Corresponding Author: Guangyi Ma. Email:
(This article belongs to the Special Issue: Intelligent Models for Security and Resilience in Cyber Physical Systems)
Computer Modeling in Engineering & Sciences 2020, 124(3), 1149-1165. https://doi.org/10.32604/cmes.2020.010932
Received 08 April 2020; Accepted 03 May 2020; Issue published 21 August 2020
Abstract
Snow cover is an important parameter in the fields of computer modeling, engineering technology and energy development. With the extensive growth of novel hardware and software compositions creating smart, cyber physical systems’ (CPS) efficient end-to-end workflows. In order to provide accurate snow detection results for the CPS’s terminal, this paper proposed a snow cover detection algorithm based on the unsupervised Gaussian mixture model (GMM) for the FY-4A satellite data. At present, most snow cover detection algorithms mainly utilize the characteristics of the optical spectrum, which is based on the normalized difference snow index (NDSI) with thresholds in different wavebands. These algorithms require a large amount of manually labeled data for statistical analysis to obtain the appropriate thresholds for the study area. Consideration must be given to both the high and low elevations in the study area. It is difficult to extract all snow by a fixed threshold in mountainous and rugged terrains. In this research, we avoid relying on a manual analysis for different elevations. Therefore, an algorithm based on the GMM is proposed, integrating the threshold-based algorithm and the GMM. First, the threshold-based algorithm with transferred thresholds from other satellites’ analysis results are used to coarsely classify the surface objects. These results are then used to initialize the parameters of the GMM. Finally, the parameters of that model are updated by an expectation-maximum (EM) iteration algorithm, and the final results are outputted when the iterative conditions end. The results show that this algorithm can adjust itself to mountainous terrain with different elevations, and exhibits a better performance than the threshold-based algorithm. Compared with orbit satellites’ snow products, the accuracy of the algorithm used for FY-4A is improved by nearly 2%, and the snow detection rate is increased by nearly 6%. Moreover, compared with microwave sensors’ snow products, the accuracy is increased by nearly 3%. The validation results show that the proposed algorithm can be adapted to a complex terrain environment in mountainous areas and exhibits good performance under a transferred threshold without manually assigned labels.Keywords
Cite This Article
Citations
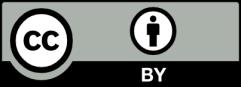