Open Access
ARTICLE
Effect of Data Augmentation of Renal Lesion Image by Nine-layer Convolutional Neural Network in Kidney CT
1 School of Education Science, Nanjing Normal University, Nanjing, 210097, China
2 Unit of Urology, Tongliao Hospital of Inner Mongolia, Tongliao, 028000, China
3 Guangxi Key Laboratory of Trusted Software, Guilin University of Electronic Technology, Guilin, 541004, China
4 School of Mathematics and Actuarial Science, University of Leicester, Leicester, LE1 7RH, UK
5 School of Architecture Building and Civil Engineering, Loughborough University, Loughborough, LE11 3TU, UK
* Corresponding Author: Shuihua Wang. Email:
(This article belongs to the Special Issue: Recent Advances on Deep Learning for Medical Signal Analysis (RADLMSA))
Computer Modeling in Engineering & Sciences 2020, 124(3), 1001-1015. https://doi.org/10.32604/cmes.2020.010753
Received 26 March 2020; Accepted 27 April 2020; Issue published 21 August 2020
Abstract
Artificial Intelligence (AI) becomes one hotspot in the field of the medical images analysis and provides rather promising solution. Although some research has been explored in smart diagnosis for the common diseases of urinary system, some problems remain unsolved completely A nine-layer Convolutional Neural Network (CNN) is proposed in this paper to classify the renal Computed Tomography (CT) images. Four group of comparative experiments prove the structure of this CNN is optimal and can achieve good performance with average accuracy about 92.07 ± 1.67%. Although our renal CT data is not very large, we do augment the training data by affine, translating, rotating and scaling geometric transformation and gamma, noise transformation in color space. Experimental results validate the Data Augmentation (DA) on training data can improve the performance of our proposed CNN compared to without DA with the average accuracy about 0.85%. This proposed algorithm gives a promising solution to help clinical doctors automatically recognize the abnormal images faster than manual judgment and more accurately than previous methods.Keywords
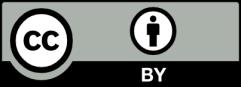