Open Access
ARTICLE
A Local Sparse Screening Identification Algorithm with Applications
1 School of Mechanical Engineering, Tianjin University, Tianjin, 300350, China
2 Tianjin Key Laboratory of Nonlinear Dynamics and Control, Tianjin, 300350, China
* Corresponding Author: Wei Wang. Email:
(This article belongs to the Special Issue: Novel Methods for Reliability Evaluation and Optimization of Complex Mechanical Structures)
Computer Modeling in Engineering & Sciences 2020, 124(2), 765-782. https://doi.org/10.32604/cmes.2020.010061
Received 10 February 2020; Accepted 11 May 2020; Issue published 20 July 2020
Abstract
Extracting nonlinear governing equations from noisy data is a central challenge in the analysis of complicated nonlinear behaviors. Despite researchers follow the sparse identification nonlinear dynamics algorithm (SINDy) rule to restore nonlinear equations, there also exist obstacles. One is the excessive dependence on empirical parameters, which increases the difficulty of data pre-processing. Another one is the coexistence of multiple coefficient vectors, which causes the optimal solution to be drowned in multiple solutions. The third one is the composition of basic function, which is exclusively applicable to specific equations. In this article, a local sparse screening identification algorithm (LSSI) is proposed to identify nonlinear systems. First, we present the k-neighbor parameter to replace all empirical parameters in data filtering. Second, we combine the mean error screening method with the SINDy algorithm to select the optimal one from multiple solutions. Third, the time variable t is introduced to expand the scope of the SINDy algorithm. Finally, the LSSI algorithm is applied to recover a classic ODE and a bi-stable energy harvester system. The results show that the new algorithm improves the ability of noise immunity and optimal parameters identification provides a desired foundation for nonlinear analyses.Keywords
Cite This Article
Citations
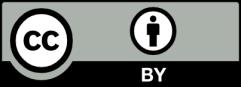