Open Access
ARTICLE
Image Information Hiding Method Based on Image Compression and Deep Neural Network
1 College of Computer and Information Engineering, Henan Normal University, Xinxiang, 453007, China.
2 School of Optical-Electrical and Computer Engineering, University of Shanghai for Science and Technology,
Shanghai, 200093, China.
* Corresponding Author: Xintao Duan. Email: .
(This article belongs to the Special Issue: Information Hiding and Multimedia Security)
Computer Modeling in Engineering & Sciences 2020, 124(2), 721-745. https://doi.org/10.32604/cmes.2020.09463
Received 17 December 2019; Accepted 23 March 2020; Issue published 20 July 2020
Abstract
Image steganography is a technique that hides secret information into the cover image to protect information security. The current image steganography is mainly to embed a smaller secret image in an area such as a texture of a larger-sized cover image, which will cause the size of the secret image to be much smaller than the cover image. Therefore, the problem of small steganographic capacity needs to be solved urgently. This paper proposes a steganography framework that combines image compression. In this framework, the Vector Quantized Variational AutoEncoder (VQ-VAE) is used to achieve the compression of the secret image. The compressed and reconstructed image is visually indistinguishable from the original image and facilitates more embedded data information later. Finally, the compressed image is transmitted to a SegNet deep neural network that contains a set of encoders and decoders to achieve image hiding and extraction. Experimental results show that the steganographic framework guarantees the quality of steganography while its relative steganographic capacity reaches 1. Besides, Peak Signal-to-Noise Ratio (PSNR) and Structural Similarity Index (SSIM) values can reach 42 dB and 0.94, respectively.Keywords
Cite This Article
Citations
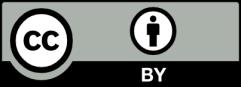
This work is licensed under a Creative Commons Attribution 4.0 International License , which permits unrestricted use, distribution, and reproduction in any medium, provided the original work is properly cited.