Open Access
ARTICLE
Investigation of Granite Deformation Process under Axial Load Using LSTM-Based Architectures
1 Department of Civil Engineering, Shanghai University, Shanghai, 200444, China
2 Institue for the Conservation of Cultural Heritage, Shanghai University, Shanghai, 200444, China
3 Department of Mining Engineering, Hamedan University of Technology, Hamedan, 65155579, Iran
4 China Railway 17 Bureau Group Co., Ltd., Taiyuan, 030006, China
* Corresponding Author: Jinming Xu. Email:
(This article belongs to the Special Issue: Machine Learning based Methods for Mechanics)
Computer Modeling in Engineering & Sciences 2020, 124(2), 643-664. https://doi.org/10.32604/cmes.2020.09866
Received 22 January 2020; Accepted 01 April 2020; Issue published 20 July 2020
Abstract
Granite is generally composed of quartz, biotite, feldspar, and cracks. The changes in digital parameters of these compositions reflect the detail of the deformation process of the rock. Therefore, the estimation of the changes in digital parameters of the compositions is much helpful to understand the deformation and failure stages of the rock. In the current study, after dividing the frames in the video images photographed during the axial compression test into four parts (or, the upper left, upper right, lower left, and lower right ones), the digital parameters of various compositions in each part were then extracted. Using these parameters as input dataset, a long short-term memory (LSTM) based neural network was then established for exploring the changes of various compositions. After dividing the deformation process into four stages based on the stress-strain curve and using the digital parameters of various compositions as the dataset, the LSTM-based neural network for estimating the rock deformation stage was also established. The root mean squared error (RMSE) and goodness of fit (R2 ) and the average accuracy (ACC) were used to evaluate the efficiencies of these two LSTM-based neural networks. The influences of variables (such as the number of hidden layers, maximum epoch, learning rate, minimum batch size and train ratio) on efficiencies of the LSTM-based neural networks were thereafter explored. It shows that the super parameters have a great influence on the efficiency of the established LSTM-based neural network for estimating digital parameter changes of various compositions; the estimations were relatively good if the number of hidden layers, maximum epoch, learning ratio, minimum batch size, and train ratio is 2, 150, 0.005, 10, and 0.8, respectively; the compositions with the greater percentage have a greater accuracy using the neural network; the great-small sequence of ACC is biotite, feldspar, crack, and quartz, if the LSTM-based architecture for estimating deformation stages was used. These results may be referable both for investigating the availably of the established LSTM-based architectures and for exploring the deformation process of the rock materials.Keywords
Cite This Article
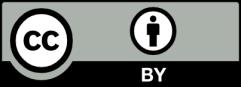
This work is licensed under a Creative Commons Attribution 4.0 International License , which permits unrestricted use, distribution, and reproduction in any medium, provided the original work is properly cited.