Open Access
ARTICLE
An Intelligent Predictive Model-Based Multi-Response Optimization of EDM Process
1 Department of Computer Science and Engineering, Vel Tech Multi Tech Dr. Rangarajan Dr. Sakunthala Engineering College, Chennai, 600062, India
2 Department of Mechanical Engineering, Sikkim Manipal Institute of Technology, Sikkim Manipal University, Majhitar, 737136, India
3 Department of Electrical and Electronics Engineering, Sikkim Manipal Institute of Technology, Sikkim Manipal University, Majhitar, 737136, India
4 Department of Mechanical Engineering, Vel Tech Rangarajan Dr. Sagunthala R&D Institute of Science and Technology, Avadi, 600062, India
5 School of Computing, University of Eastern Finland, Kuopio, 70211, Finland
* Corresponding Author: K. Kalita. Email:
Computer Modeling in Engineering & Sciences 2020, 124(2), 459-476. https://doi.org/10.32604/cmes.2020.09645
Received 12 January 2020; Accepted 07 May 2020; Issue published 20 July 2020
Abstract
Electrical Discharge Machining (EDM) is a popular non-traditional machining process that is widely used due to its ability to machine hard and brittle materials. It does not require a cutting tool and can machine complex geometries easily. However, it suffers from drawbacks like a poor rate of machining and excessive tool wear. In this research, an attempt is made to address these issues by using an intelligent predictive model coupled global optimization approach to predict suitable combinations of input parameters (current, pulse on-time and pulse off-time) that would effectively increase the material removal rate and minimize the tool wear. The predictive models, which are based on the symbolic regression approach exploit the machine intelligence of Genetic Programming (GP). As compared to traditional polynomial response surface (PRS) predictive models, the GP predictive models show compactness as well as better prediction capability. The developed GP predictive models are deployed in conjunction with NSGA-II to predict Pareto optimal solutions.Keywords
Cite This Article
Citations
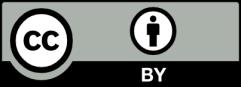
This work is licensed under a Creative Commons Attribution 4.0 International License , which permits unrestricted use, distribution, and reproduction in any medium, provided the original work is properly cited.