Open Access
ARTICLE
A Re-Parametrization-Based Bayesian Differential Analysis Algorithm for Gene Regulatory Networks Modeled with Structural Equation Models
1 College of Computer Science and Technology, Jilin University, Changchun, 130012, China
2 Key Laboratory of Symbolic Computation and Knowledge Engineering of Ministry of Education, Jilin University, Changchun, 130012, China
* Corresponding Author: Jie Liu. Email:
(This article belongs to the Special Issue: Data Science and Modeling in Biology, Health, and Medicine)
Computer Modeling in Engineering & Sciences 2020, 124(1), 303-313. https://doi.org/10.32604/cmes.2020.09353
Received 05 December 2019; Accepted 20 March 2020; Issue published 19 June 2020
Abstract
Under different conditions, gene regulatory networks (GRNs) of the same gene set could be similar but different. The differential analysis of GRNs under different conditions is important for understanding condition-specific gene regulatory relationships. In a naive approach, existing GRN inference algorithms can be used to separately estimate two GRNs under different conditions and identify the differences between them. However, in this way, the similarities between the pairwise GRNs are not taken into account. Several joint differential analysis algorithms have been proposed recently, which were proved to outperform the naive approach apparently. In this paper, we model the GRNs under different conditions with structural equation models (SEMs) to integrate gene expression data and genetic perturbations, and re-parameterize the pairwise SEMs to form an integrated model that incorporates the differential structure. Then, a Bayesian inference method is used to make joint differential analysis by solving the integrated model. We evaluated the performance of the proposed re-parametrization-based Bayesian differential analysis (ReBDA) algorithm by running simulations on synthetic data with different settings. The performance of the ReBDA algorithm was demonstrated better than another state-of-the-art joint differential analysis algorithm for SEMs ReDNet obviously. In the end, the ReBDA algorithm was applied to make differential analysis on a real human lung gene data set to illustrate its applicability and practicability.Keywords
Cite This Article
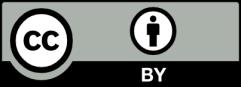