Open Access
ARTICLE
An Improved Non-Parametric Method for Multiple Moving Objects Detection in the Markov Random Field
1 College of Electric and Information Engineering, Hunan Institute of Engineering, Xiangtan, 411104, China
2 National Engineering Research Laboratory for Robot Vision Perception and Control, Hunan University, Changsha, China
3 The Laboratory for Computational Neuroscience, University of Pittsburgh, Pittsburgh, PA 15260, USA
* Corresponding Author: Qin Wan. Email:
Computer Modeling in Engineering & Sciences 2020, 124(1), 129-149. https://doi.org/10.32604/cmes.2020.09397
Received 10 December 2019; Accepted 01 April 2020; Issue published 19 June 2020
Abstract
Detecting moving objects in the stationary background is an important problem in visual surveillance systems. However, the traditional background subtraction method fails when the background is not completely stationary and involves certain dynamic changes. In this paper, according to the basic steps of the background subtraction method, a novel non-parametric moving object detection method is proposed based on an improved ant colony algorithm by using the Markov random field. Concretely, the contributions are as follows: 1) A new nonparametric strategy is utilized to model the background, based on an improved kernel density estimation; this approach uses an adaptive bandwidth, and the fused features combine the colours, gradients and positions. 2) A Markov random field method based on this adaptive background model via the constraint of the spatial context is proposed to extract objects. 3) The posterior function is maximized efficiently by using an improved ant colony system algorithm. Extensive experiments show that the proposed method demonstrates a better performance than many existing state-of-the-art methods.Keywords
Cite This Article
Citations
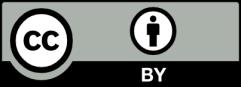
This work is licensed under a Creative Commons Attribution 4.0 International License , which permits unrestricted use, distribution, and reproduction in any medium, provided the original work is properly cited.