Open Access
ARTICLE
Machine Learning Model Comparison for Automatic Segmentation of Intracoronary Optical Coherence Tomography and Plaque Cap Thickness Quantification
1 School of Biological Science and Medical Engineering, Southeast University, Nanjing, 210096, China
2 School of Information Science and Engineering, Yunnan University, Kunming, 650091, China
3 School of Science, Nanjing University of Posts and Telecommunications, Nanjing, 210023, China
4 Department of Medicine, Emory University School of Medicine, Atlanta, GA, 30307, USA
5 The Wallace H. Coulter Department of Biomedical Engineering, Georgia Institute of Technology, Atlanta, GA, 30332, USA
6 Institute for Medical Engineering and Science, Massachusetts Institute of Technology, Cambridge, MA, 02139, USA
7 Mathematical Sciences Department, Worcester Polytechnic Institute, Worcester, MA 01609, USA
8 School of Mathematics, Southeast University, Nanjing, 210096, China
* Corresponding Authors: Dalin Tang, Southeast University. Email: ; Rencan Nie. Email:
Computer Modeling in Engineering & Sciences 2020, 123(2), 631-646. https://doi.org/10.32604/cmes.2020.09718
Received 19 January 2020; Accepted 06 March 2020; Issue published 01 May 2020
Abstract
Optical coherence tomography (OCT) is a new intravascular imaging technique with high resolution and could provide accurate morphological information for plaques in coronary arteries. However, its segmentation is still commonly performed manually by experts which is time-consuming. The aim of this study was to develop automatic techniques to characterize plaque components and quantify plaque cap thickness using 3 machine learning methods including convolutional neural network (CNN) with U-Net architecture, CNN with Fully convolutional DenseNet (FC-DenseNet) architecture and support vector machine (SVM). In vivo OCT and intravascular ultrasound (IVUS) images were acquired from two patients at Emory University with informed consent obtained. Eighteen OCT image slices which included lipid core and with acceptable image quality were selected for our study. Manual segmentation from imaging experts was used as the gold standard for model training and validation. Since OCT has limited penetration, virtual histology IVUS was combined with OCT data to improve reliability. A 3-fold cross-validation method was used for model training and validation. The overall tissue classification accuracy for the 18 slices studied (total classification database sample size was 8580096 pixels) was 96.36% and 92.72% for U-Net and FC-DenseNet, respectively. The best average prediction accuracy for lipid was 91.29% based on SVM, compared to 82.84% and 78.91% from U-Net and FC-DenseNet, respectively. The overall average accuracy (Acc) differentiating lipid and fibrous tissue were 95.58%, 92.33% and 81.84% for U-Net, FC-DenseNet and SVM, respectively. The average errors of U-Net, FC-DenseNet and SVM from the 18 slices for cap thickness quantification were 8.83%, 10.71% and 15.85%. The average relative errors of minimum cap thickness from 18 slices of U-Net, FC-DenseNet and SVM were 17.46%, 13.06% This work is licensed under a Creative Commons Attribution 4.0 International License, which permits unrestricted use, distribution, and reproduction in any medium, provided the original work is properly cited. Computer Modeling in Engineering & Sciences DOI:10.32604/cmes.2020.09718 Article Tech Science Press and 22.20%, respectively. To conclude, CNN-based segmentation methods can better characterize plaque compositions and quantify plaque cap thickness on OCT images and are more likely to be used in the clinical arena. Large-scale studies are needed to further develop the methods and validate our findings.Keywords
Cite This Article
Citations
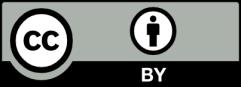