Open Access
ARTICLE
Simulation of Daily Diffuse Solar Radiation Based on Three Machine Learning Models
1 Faculty of Agriculture and Food, Kunming University of Science and Technology, Kunming, 650500, China.
2 School of Hydraulic and Ecological Engineering, Nanchang Institute of Technology, Nanchang, 330099, China.
3 Jiangxi Key Laboratory of Hydrology-Water Resources and Water Environment, Nanchang Institute of Technology, Nanchang, 330099, China.
* Corresponding Author: Xiaogang Liu; Email: .
Computer Modeling in Engineering & Sciences 2020, 123(1), 49-73. https://doi.org/10.32604/cmes.2020.09014
Received 02 November 2019; Accepted 19 December 2019; Issue published 01 April 2020
Abstract
Solar radiation is an important parameter in the fields of computer modeling, engineering technology and energy development. This paper evaluated the ability of three machine learning models, i.e., Extreme Gradient Boosting (XGBoost), Support Vector Machine (SVM) and Multivariate Adaptive Regression Splines (MARS), to estimate the daily diffuse solar radiation (Rd). The regular meteorological data of 1966-2015 at five stations in China were taken as the input parameters (including mean average temperature (Ta), theoretical sunshine duration (N), actual sunshine duration (n), daily average air relative humidity (RH), and extra-terrestrial solar radiation (Ra)). And their estimation accuracies were subjected to comparative analysis. The three models were first trained using meteorological data from 1966 to 2000. Then, the 2001-2015 data was used to test the trained machine learning model. The results show that the XGBoost had better accuracy than the other two models in coefficient of determination (R2 ), root mean square error (RMSE), mean bias error (MBE) and normalized root mean square error (NRMSE). The MARS performed better in the training phase than the testing phase, but became less accurate in the testing phase, with the R2 value falling by 2.7-16.9% on average. By contrast, the R2 values of SVM and XGBoost increased by 2.9-12.2% and 1.9-14.3%, respectively. Despite trailing slightly behind the SVM at the Beijing station, the XGBoost showed good performance at the rest of the stations in the two phases. In the training phase, the accuracy growth is small but observable. In addition, the XGBoost had a slightly lower RMSE than the SVM, a signal of its edge in stability. Therefore, the three machine learning models can estimate the daily Rd based on local inputs and the XGBoost stands out for its excellent performance and stability.Keywords
Cite This Article
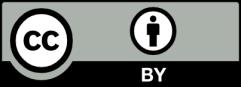
This work is licensed under a Creative Commons Attribution 4.0 International License , which permits unrestricted use, distribution, and reproduction in any medium, provided the original work is properly cited.