Open Access
ARTICLE
Growing and Pruning Based Deep Neural Networks Modeling for Effective Parkinson’s Disease Diagnosis
1 Kastamonu University, Kuzeykent Yerleşkesi, Kastamonu, 37100, Turkey.
* Corresponding Author: Kemal Akyol. Email: kakyol@kastamonu.edu.tr.
(This article belongs to the Special Issue: Data Science and Modeling in Biology, Health, and Medicine)
Computer Modeling in Engineering & Sciences 2020, 122(2), 619-632. https://doi.org/10.32604/cmes.2020.07632
Received 13 June 2019; Accepted 18 September 2019; Issue published 01 February 2020
Abstract
Parkinson’s disease is a serious disease that causes death. Recently, a new dataset has been introduced on this disease. The aim of this study is to improve the predictive performance of the model designed for Parkinson’s disease diagnosis. By and large, original DNN models were designed by using specific or random number of neurons and layers. This study analyzed the effects of parameters, i.e., neuron number and activation function on the model performance based on growing and pruning approach. In other words, this study addressed the optimum hidden layer and neuron numbers and ideal activation and optimization functions in order to find out the best Deep Neural Networks model. In this context of this study, several models were designed and evaluated. The overall results revealed that the Deep Neural Networks were significantly successful with 99.34% accuracy value on test data. Also, it presents the highest prediction performance reported so far. Therefore, this study presents a model promising with respect to more accurate Parkinson’s disease diagnosis.Keywords
Cite This Article
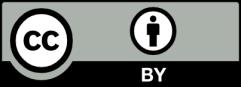
This work is licensed under a Creative Commons Attribution 4.0 International License , which permits unrestricted use, distribution, and reproduction in any medium, provided the original work is properly cited.