Open Access
REVIEW
Wind Power Forecasting Methods Based on Deep Learning: A Survey
1 School of Computer Science, Jiangsu University of Science and Technology, Zhenjiang, China.
2 School of Automation, Key Laboratory of Measurement and Control for CSE, Ministry of Education, Southeast University, Nanjing, China.
3 Department of Electrical and Computer Engineering, University of Nevada, Las Vegas, USA.
* Corresponding Author: Haijian Shao. Email: .
Computer Modeling in Engineering & Sciences 2020, 122(1), 273-301. https://doi.org/10.32604/cmes.2020.08768
Received 07 October 2019; Accepted 16 December 2019; Issue published 01 January 2020
Abstract
Accurate wind power forecasting in wind farm can effectively reduce the enormous impact on grid operation safety when high permeability intermittent power supply is connected to the power grid. Aiming to provide reference strategies for relevant researchers as well as practical applications, this paper attempts to provide the literature investigation and methods analysis of deep learning, enforcement learning and transfer learning in wind speed and wind power forecasting modeling. Usually, wind speed and wind power forecasting around a wind farm requires the calculation of the next moment of the definite state, which is usually achieved based on the state of the atmosphere that encompasses nearby atmospheric pressure, temperature, roughness, and obstacles. As an effective method of high-dimensional feature extraction, deep neural network can theoretically deal with arbitrary nonlinear transformation through proper structural design, such as adding noise to outputs, evolutionary learning used to optimize hidden layer weights, optimize the objective function so as to save information that can improve the output accuracy while filter out the irrelevant or less affected information for forecasting. The establishment of high-precision wind speed and wind power forecasting models is always a challenge due to the randomness, instantaneity and seasonal characteristics.Keywords
Cite This Article
Citations
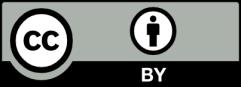
This work is licensed under a Creative Commons Attribution 4.0 International License , which permits unrestricted use, distribution, and reproduction in any medium, provided the original work is properly cited.