Open Access
ARTICLE
IDSH: An Improved Deep Supervised Hashing Method for Image Retrieval
1 School of Optical-Electrical and Computer Engineering, University of Shanghai for Science and Technology, Shanghai, 200093, China.
2 Major of Electrical Engineering and Electronics, Graduate School of Engineering, Kogakuin University, 1-24-2, Nishi-shinjuku, Sinjuku-ku, Tokyo, 163-8677, Japan.
a Both authors contributed equally to this work.
∗ Corresponding Authors: Feifei Lee. Email: ;
Qiu Chen. Email: .
Computer Modeling in Engineering & Sciences 2019, 121(2), 593-608. https://doi.org/10.32604/cmes.2019.07796
Abstract
Image retrieval has become more and more important because of the explosive growth of images on the Internet. Traditional image retrieval methods have limited image retrieval performance due to the poor image expression abhility of visual feature and high dimension of feature. Hashing is a widely-used method for Approximate Nearest Neighbor (ANN) search due to its rapidity and timeliness. Meanwhile, Convolutional Neural Networks (CNNs) have strong discriminative characteristics which are used for image classification. In this paper, we propose a CNN architecture based on improved deep supervised hashing (IDSH) method, by which the binary compact codes can be generated directly. The main contributions of this paper are as follows: first, we add a Batch Normalization (BN) layer before each activation layer to prevent the gradient from vanishing and improve the training speed; secondly, we use Divide-and-Encode Module to map image features to approximate hash codes; finally, we adopt center loss to optimize training. Extensive experimental results on four large-scale datasets: MNIST, CIFAR-10, NUS-WIDE and SVHN demonstrate the effectiveness of the proposed method compared with other state-of-the-art hashing methods.Keywords
Cite This Article
Citations
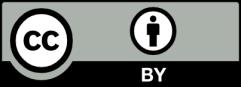
This work is licensed under a Creative Commons Attribution 4.0 International License , which permits unrestricted use, distribution, and reproduction in any medium, provided the original work is properly cited.