Open Access
ARTICLE
Novel Ensemble Modeling Method for Enhancing Subset Diversity Using Clustering Indicator Vector Based on Stacked Autoencoder
1 Key Laboratory of Advanced Control and Optimization for Chemical Processes of Ministry of Education, East China University of Science and Technology, Shanghai, 200237, China.
* Corresponding Author: Xuefeng Yan. Email: xfyan@ecust.edu.cn.
Computer Modeling in Engineering & Sciences 2019, 121(1), 123-144. https://doi.org/10.32604/cmes.2019.07052
Abstract
A single model cannot satisfy the high-precision prediction requirements given the high nonlinearity between variables. By contrast, ensemble models can effectively solve this problem. Three key factors for improving the accuracy of ensemble models are namely the high accuracy of a submodel, the diversity between subsample sets and the optimal ensemble method. This study presents an improved ensemble modeling method to improve the prediction precision and generalization capability of the model. Our proposed method first uses a bagging algorithm to generate multiple subsample sets. Second, an indicator vector is defined to describe these subsample sets. Third, subsample sets are selected on the basis of the results of agglomerative nesting clustering on indicator vectors to maximize the diversity between subsets. Subsequently, these subsample sets are placed in a stacked autoencoder for training. Finally, XGBoost algorithm, rather than the traditional simple average ensemble method, is imported to ensemble the model during modeling. Three machine learning public datasets and atmospheric column dry point dataset from a practical industrial process show that our proposed method demonstrates high precision and improved prediction ability.Keywords
Cite This Article
Citations
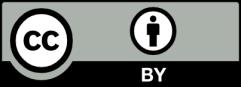
This work is licensed under a Creative Commons Attribution 4.0 International License , which permits unrestricted use, distribution, and reproduction in any medium, provided the original work is properly cited.