Open Access
ARTICLE
Optimization of Well Position and Sampling Frequency for Groundwater Monitoring and Inverse Identification of Contamination Source Conditions Using Bayes’ Theorem
School of Environment Science and Spatial Informatics, China University of Mining and Technology, Xuzhou, 221116, China.
School of Mathematics, China University of Mining and Technology, Xuzhou, 221116, China.
GHD Services, Inc, 455 Philip Street, Waterloo, Ontario, Canada.
Division of Operation and Maintenance Engineering, Department of Civil, Environmental and Natural Resources Engineering, Luleå University of Technology, 97187 Luleå, Sweden.
Xuzhou Urban Water Resources Management Office, Xuzhou, 221018, China.
* Corresponding Authors: Jing Qiang. Email: ;
Hongze Gao. Email: .
(This article belongs to the Special Issue: Beyond the Hypes of Geospatial Big Data: Theories, Methods, Analytics, and Applications)
Computer Modeling in Engineering & Sciences 2019, 119(2), 373-394. https://doi.org/10.32604/cmes.2019.03825
Abstract
Coupling Bayes’ Theorem with a two-dimensional (2D) groundwater solute advection-diffusion transport equation allows an inverse model to be established to identify a set of contamination source parameters including source intensity (M ), release location ( X0 , Y0) and release time (T0), based on monitoring well data. To address the issues of insufficient monitoring wells or weak correlation between monitoring data and model parameters, a monitoring well design optimization approach was developed based on the Bayesian formula and information entropy. To demonstrate how the model works, an exemplar problem with an instantaneous release of a contaminant in a confined groundwater aquifer was employed. The information entropy of the model parameters posterior distribution was used as a criterion to evaluate the monitoring data quantity index. The optimal monitoring well position and monitoring frequency were solved by the two-step Monte Carlo method and differential evolution algorithm given a known well monitoring locations and monitoring events. Based on the optimized monitoring well position and sampling frequency, the contamination source was identified by an improved Metropolis algorithm using the Latin hypercube sampling approach. The case study results show that the following parameters were obtained: 1) the optimal monitoring well position (D) is at (445, 200); and 2) the optimal monitoring frequency (Δt) is 7, providing that the monitoring events is set as 5 times. Employing the optimized monitoring well position and frequency, the mean errors of inverse modeling results in source parameters (M, X0, Y0, T0) were 9.20%, 0.25%, 0.0061%, and 0.33%, respectively. The optimized monitoring well position and sampling frequency can effectively safeguard the inverse modeling results in identifying the contamination source parameters. It was also learnt that the improved Metropolis-Hastings algorithm (a Markov chain Monte Carlo method) can make the inverse modeling result independent of the initial sampling points and achieves an overall optimization, which significantly improved the accuracy and numerical stability of the inverse modeling results.Keywords
Cite This Article
Citations
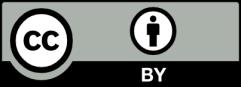
This work is licensed under a Creative Commons Attribution 4.0 International License , which permits unrestricted use, distribution, and reproduction in any medium, provided the original work is properly cited.