Open Access
ARTICLE
Gender-Specific Multi-Task Micro-Expression Recognition Using Pyramid CGBP-TOP Feature
School of Computer Science and Engineering, Jiangsu University of Science and Technology, Zhenjiang, 212003, China.
School of Computer Science and Technology, Chongqing University of Posts and Telecommunications, Chongqing, 400065, China.
* Corresponding Author: Chunlong Hu. Email: .
Computer Modeling in Engineering & Sciences 2019, 118(3), 547-559. https://doi.org/10.31614/cmes.2019.04032
Abstract
Micro-expression recognition has attracted growing research interests in the field of compute vision. However, micro-expression usually lasts a few seconds, thus it is difficult to detect. This paper presents a new framework to recognize micro-expression using pyramid histogram of Centralized Gabor Binary Pattern from Three Orthogonal Panels (CGBP-TOP) which is an extension of Local Gabor Binary Pattern from Three Orthogonal Panels feature. CGBP-TOP performs spatial and temporal analysis to capture the local facial characteristics of micro-expression image sequences. In order to keep more local information of the face, CGBP-TOP is extracted based on pyramid sub-regions of the micro-expression video frame. The combination of CGBP-TOP and spatial pyramid can represent well and truly the facial movements of the micro-expression image sequences. However, the dimension of our pyramid CGBP-TOP tends to be very high, which may lead to high data redundancy problem. In addition, it is clear that people of different genders usually have different ways of micro-expression. Therefore, in this paper, in order to select the relevant features of micro-expression, the gender-specific sparse multi-task learning method with adaptive regularization term is adopted to learn a compact subset of pyramid CGBP-TOP feature for micro-expression classification of different sexes. Finally, extensive experiments on widely used CASME II and SMIC databases demonstrate that our method can efficiently extract micro-expression motion features in the micro-expression video clip. Moreover, our proposed approach achieves comparable results with the state-of-the-art methods.Keywords
Cite This Article
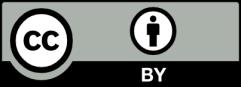
This work is licensed under a Creative Commons Attribution 4.0 International License , which permits unrestricted use, distribution, and reproduction in any medium, provided the original work is properly cited.