Open Access
ARTICLE
Nonlinear Activation Functions in CNN Based on Fluid Dynamics and Its Applications
Department of Mathematical Information Engineering, College of Industrial Technology, Nihon University,
Chiba 275-8575, Japan.
Department of Liberal Arts and Basic Sciences, College of Industrial Technology, Nihon University, Chiba
275-8576, Japan.
* Corresponding Author: Kazuhiko Kakuda. Email: .
Computer Modeling in Engineering & Sciences 2019, 118(1), 1-14. https://doi.org/10.31614/cmes.2019.04676
Abstract
The nonlinear activation functions in the deep CNN (Convolutional Neural Network) based on fluid dynamics are presented. We propose two types of activation functions by applying the so-called parametric softsign to the negative region. We use significantly the well-known TensorFlow as the deep learning framework. The CNN architecture consists of three convolutional layers with the max-pooling and one fully-connected softmax layer. The CNN approaches are applied to three benchmark datasets, namely, MNIST, CIFAR-10, and CIFAR-100. Numerical results demonstrate the workability and the validity of the present approach through comparison with other numerical performances.Keywords
Cite This Article
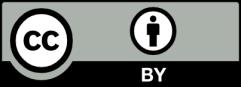
This work is licensed under a Creative Commons Attribution 4.0 International License , which permits unrestricted use, distribution, and reproduction in any medium, provided the original work is properly cited.