Open Access
ARTICLE
Estimating the Properties of Ground-Waste-Brick Mortars Using DNN and ANN
Department of Computer Engineering, Kastamonu University, Kastamonu, Turkey.
Department of Civil Engineering, Kastamonu University, Kastamonu, Turkey.
Department of Information Systems Engineering, Muğla Sıtkı Koçman University, Turkey.
Department of Civil Engineering, Kırıkkale University, Kırıkkale, Turkey.
Department of Civil Engineering, Gazi University, Ankara, Turkey.
* Corresponding Author: Abdulkadir Karaci. Email: .
Computer Modeling in Engineering & Sciences 2019, 118(1), 207-228. https://doi.org/10.31614/cmes.2019.04216
Abstract
In this study, deep-neural-network (DNN)- and artificial-neural-network (ANN)-based models along with regression models have been developed to estimate the pressure, bending and elongation values of ground-brick (GB)-added mortar samples. This study is aimed at utilizing GB as a mineral additive in concrete in the ratios 0.0%, 2.5%, 5.0%, 7.5%, 10.0%, 12.5% and 15.0%. In this study, 756 mortar samples were produced for 84 different series and were cured in tap water (W), 5% sodium sulphate solution (SS5) and 5% ammonium nitrate solution (AN5) for 7 days, 28 days, 90 days and 180 days. The developed DNN models have three inputs and two hidden layers with 20 neurons and one output, whereas the ANN models have three inputs, one output and one hidden layer with 15 neurons. Twenty-five previously obtained experimental sample datasets were used to train these developed models and to generate the regression equation. Fifty-nine non-training-attributed datasets were used to test the models. When these test values were attributed to the trained DNN, ANN and regression models, the brick-dust pressure as well as the bending and elongation values have been observed to be very close to the experimental values. Although only a small fraction (30%) of the experimental data were used for training, both the models performed the estimation process at a level that was in accordance with the opinions of experts. The fact that this success has been achieved using very little training data shows that the models have been appropriately designed. In addition, the DNN models exhibited better performance as compared with that exhibited by the ANN models. The regression model is a model whose performance is worst and unacceptable; further, the prediction error is observed to be considerably high. In conclusion, ANN- and DNN-based models are practical and effective to estimate these values.Keywords
Cite This Article
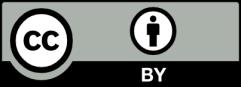
This work is licensed under a Creative Commons Attribution 4.0 International License , which permits unrestricted use, distribution, and reproduction in any medium, provided the original work is properly cited.