Open Access
ARTICLE
A Deep Learning-Based Computational Algorithm for Identifying Damage Load Condition: An Artificial Intelligence Inverse Problem Solution for Failure Analysis
College of Shipbuilding Engineering, Harbin Engineering University, Harbin, China.
Department of Civil and Environmental Engineering, University of California, Berkeley, CA,
USA.
* Corresponding Author: Shaofan Li. Email:
(This article belongs to the Special Issue: Data-driven Computational Modeling and Simulations)
Computer Modeling in Engineering & Sciences 2018, 117(3), 287-307. https://doi.org/10.31614/cmes.2018.04697
Abstract
In this work, we have developed a novel machine (deep) learning computational framework to determine and identify damage loading parameters (conditions) for structures and materials based on the permanent or residual plastic deformation distribution or damage state of the structure. We have shown that the developed machine learning algorithm can accurately and (practically) uniquely identify both prior static as well as impact loading conditions in an inverse manner, based on the residual plastic strain and plastic deformation as forensic signatures. The paper presents the detailed machine learning algorithm, data acquisition and learning processes, and validation/verification examples. This development may have significant impacts on forensic material analysis and structure failure analysis, and it provides a powerful tool for material and structure forensic diagnosis, determination, and identification of damage loading conditions in accidental failure events, such as car crashes and infrastructure or building structure collapses.Keywords
Cite This Article
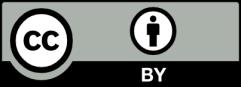
This work is licensed under a Creative Commons Attribution 4.0 International License , which permits unrestricted use, distribution, and reproduction in any medium, provided the original work is properly cited.