Open Access
ARTICLE
Online Group Recommendation with Local Optimization
School of Computer Science and Engineering, Jiangsu University of Science and Technology, Zhenjiang, China.
*Corresponding Author: Haitao Zou. Email: .
Computer Modeling in Engineering & Sciences 2018, 115(2), 217-231. https://doi.org/10.3970/cmes.2018.00194
Abstract
There are some scenarios that need group recommendation such as watching a movie or a TV series, selecting a tourist destination, or having dinner together. Approaches in this domain can be divided into two categories: Creating group profiles and aggregating individual recommender list. Yet none of the above methods can handle the online group recommendation both efficiently and accurately and these methods either strongly limited by their application environment, or bring bias towards those users having limited connections with this group. In this work, we propose a local optimization framework, using sub-group profiles to compute the item relevance. Such method can captures and removes the bias existed in the traditional group recommendation algorithms in a certain degree. It can then be used to derive single-user recommendation. We also propose an approach to overcome the problem caused by dynamic change or user updating about his social network, which detects the target user’s group by analyzing the link types between he and his neighbours, and then use the group information to generate his recommendations. Experimental analysis for group and personal recommendation on three different sizes of MovieLens datasets show fairly good results, our method consistently outperform several state-of-the-arts in efficiency. And we also provide the explanations behind the phenomena during the experiments.Keywords
Cite This Article
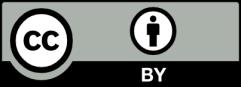