Open Access
ARTICLE
Intrusion Detection in NSL-KDD Dataset Using Hybrid Self-Organizing Map Model
1 Knowledge Unit of Systems and Technology, University of Management and Technology, Sialkot, 51310, Pakistan
2 Department of Computer Science, University of Wah, Wah Cantt, 47010, Pakistan
3 Department of Computer Engineering, College of Computer and Information Sciences (CCIS), King Saud University, Riyadh, 11451, Saudi Arabia
4 Department of AI Convergence Network, Ajou University, Suwon, 16499, Republic of Korea
* Corresponding Author: Jehad Ali. Email:
(This article belongs to the Special Issue: Leveraging AI and ML for QoS Improvement in Intelligent Programmable Networks)
Computer Modeling in Engineering & Sciences 2025, 143(1), 639-671. https://doi.org/10.32604/cmes.2025.062788
Received 27 December 2024; Accepted 18 February 2025; Issue published 11 April 2025
Abstract
Intrusion attempts against Internet of Things (IoT) devices have significantly increased in the last few years. These devices are now easy targets for hackers because of their built-in security flaws. Combining a Self-Organizing Map (SOM) hybrid anomaly detection system for dimensionality reduction with the inherited nature of clustering and Extreme Gradient Boosting (XGBoost) for multi-class classification can improve network traffic intrusion detection. The proposed model is evaluated on the NSL-KDD dataset. The hybrid approach outperforms the baseline line models, Multilayer perceptron model, and SOM-KNN (k-nearest neighbors) model in precision, recall, and F1-score, highlighting the proposed approach’s scalability, potential, adaptability, and real-world applicability. Therefore, this paper proposes a highly efficient deployment strategy for resource-constrained network edges. The results reveal that Precision, Recall, and F1-scores rise 10%–30% for the benign, probing, and Denial of Service (DoS) classes. In particular, the DoS, probe, and benign classes improved their F1-scores by 7.91%, 32.62%, and 12.45%, respectively.Keywords
Cite This Article
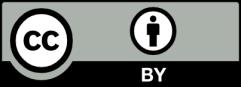
This work is licensed under a Creative Commons Attribution 4.0 International License , which permits unrestricted use, distribution, and reproduction in any medium, provided the original work is properly cited.