Open Access
ARTICLE
MOCBOA: Multi-Objective Chef-Based Optimization Algorithm Using Hybrid Dominance Relations for Solving Engineering Design Problems
1 Computer Science Department, University of M’sila, Ichebilia, M’sila, 28000, Algeria
2 Computer Science Department, Mohamed El Bachir El Ibrahimi University of Bordj BouArreridj, Bordj BouArreridj, 34000, Algeria
3 Department of Computer Engineering, College of Computer and Information Sciences, King Saud University, Riyadh, 11543, Saudi Arabia
4 Operations Research Department, Faculty of Graduate Studies for Statistical Research, Cairo University, Giza, 12613, Egypt
5 Applied Science Research Center, Applied Science Private University, Amman, 11931, Jordan
6 Faculty of Mathematics, Otto-von-Guericke University, Magdeburg, 39016, Germany
7 University Centre for Research and Development, Chandigarh University, Mohali, 140413, India
8 Department of Electrical and Computer Engineering, Shahid Beheshti University, Tehran, 14399, Iran
9 DTU AI and Data Science Hub (DAIDASH), Duy Tan University, Da Nang, 550000, Vietnam
* Corresponding Author: Mohammad Shokouhifar. Email:
(This article belongs to the Special Issue: Swarm and Metaheuristic Optimization for Applied Engineering Application)
Computer Modeling in Engineering & Sciences 2025, 143(1), 967-1008. https://doi.org/10.32604/cmes.2025.062332
Received 16 December 2024; Accepted 07 March 2025; Issue published 11 April 2025
Abstract
Multi-objective optimization is critical for problem-solving in engineering, economics, and AI. This study introduces the Multi-Objective Chef-Based Optimization Algorithm (MOCBOA), an upgraded version of the Chef-Based Optimization Algorithm (CBOA) that addresses distinct objectives. Our approach is unique in systematically examining four dominance relations—Pareto, Epsilon, Cone-epsilon, and Strengthened dominance—to evaluate their influence on sustaining solution variety and driving convergence toward the Pareto front. Our comparison investigation, which was conducted on fifty test problems from the CEC 2021 benchmark and applied to areas such as chemical engineering, mechanical design, and power systems, reveals that the dominance approach used has a considerable impact on the key optimization measures such as the hypervolume metric. This paper provides a solid foundation for determining the most effective dominance approach and significant insights for both theoretical research and practical applications in multi-objective optimization.Keywords
Cite This Article
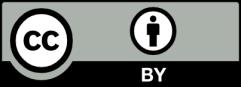
This work is licensed under a Creative Commons Attribution 4.0 International License , which permits unrestricted use, distribution, and reproduction in any medium, provided the original work is properly cited.