Open Access
ARTICLE
Coupling the Power of YOLOv9 with Transformer for Small Object Detection in Remote-Sensing Images
Department of Electrical Engineering, College of Engineering, Northern Border University, Arar, 91431, Saudi Arabia
* Corresponding Author: Mohammad Barr. Email:
(This article belongs to the Special Issue: Advances in AI-Driven Computational Modeling for Image Processing)
Computer Modeling in Engineering & Sciences 2025, 143(1), 593-616. https://doi.org/10.32604/cmes.2025.062264
Received 14 December 2024; Accepted 11 March 2025; Issue published 11 April 2025
Abstract
Recent years have seen a surge in interest in object detection on remote sensing images for applications such as surveillance and management. However, challenges like small object detection, scale variation, and the presence of closely packed objects in these images hinder accurate detection. Additionally, the motion blur effect further complicates the identification of such objects. To address these issues, we propose enhanced YOLOv9 with a transformer head (YOLOv9-TH). The model introduces an additional prediction head for detecting objects of varying sizes and swaps the original prediction heads for transformer heads to leverage self-attention mechanisms. We further improve YOLOv9-TH using several strategies, including data augmentation, multi-scale testing, multi-model integration, and the introduction of an additional classifier. The cross-stage partial (CSP) method and the ghost convolution hierarchical graph (GCHG) are combined to improve detection accuracy by better utilizing feature maps, widening the receptive field, and precisely extracting multi-scale objects. Additionally, we incorporate the E-SimAM attention mechanism to address low-resolution feature loss. Extensive experiments on the VisDrone2021 and DIOR datasets demonstrate the effectiveness of YOLOv9-TH, showing good improvement in mAP compared to the best existing methods. The YOLOv9-TH-e achieved 54.2% of mAP50 on the VisDrone2021 dataset and 92.3% of mAP on the DIOR dataset. The results confirm the model’s robustness and suitability for real-world applications, particularly for small object detection in remote sensing images.Keywords
Cite This Article
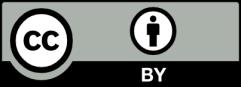
This work is licensed under a Creative Commons Attribution 4.0 International License , which permits unrestricted use, distribution, and reproduction in any medium, provided the original work is properly cited.