Open Access
ARTICLE
Parameters Estimation of Modified Triple Diode Model of PSCs Considering Charge Accumulations and Electric Field Effects Using Puma Optimizer
1 Electrical Engineering Department, Faculty of Engineering, Kafrelsheikh University, Kafrelsheikh, 33516, Egypt
2 Department of Computer Engineering and Information, College of Engineering in Wadi Alddawasir, Prince Sattam Bin Abdulaziz University, Kharj, 16278, Saudi Arabia
3 Machine Learning and Information Retrieval Department, Artificial Intelligence, Kafrelsheikh University, Kafrelsheikh, 33516, Egypt
4 Electrical Department, Faculty of Engineering and Technology, Badr University in Cairo, Badr, 11829, Egypt
5 Physics and Mathematics Department, Faculty of Engineering, Kafrelsheikh University, Kafrelsheikh, 33516, Egypt
6 College of Engineering, Al-Ayen University, Thi-Qar, 64001, Iraq
* Corresponding Author: Mona Gafar. Email:
(This article belongs to the Special Issue: Computational Intelligent Systems for Solving Complex Engineering Problems: Principles and Applications-II)
Computer Modeling in Engineering & Sciences 2025, 143(1), 723-745. https://doi.org/10.32604/cmes.2025.059625
Received 13 October 2024; Accepted 20 February 2025; Issue published 11 April 2025
Abstract
Promoting the high penetration of renewable energies like photovoltaic (PV) systems has become an urgent issue for expanding modern power grids and has accomplished several challenges compared to existing distribution grids. This study measures the effectiveness of the Puma optimizer (PO) algorithm in parameter estimation of PSC (perovskite solar cells) dynamic models with hysteresis consideration considering the electric field effects on operation. The models used in this study will incorporate hysteresis effects to capture the time-dependent behavior of PSCs accurately. The PO optimizes the proposed modified triple diode model (TDM) with a variable voltage capacitor and resistances (VVCARs) considering the hysteresis behavior. The suggested PO algorithm contrasts with other well-known optimizers from the literature to demonstrate its superiority. The results emphasize that the PO realizes a lower RMSE (Root mean square errors), which proves its capability and efficacy in parameter extraction for the models. The statistical results emphasize the efficiency and supremacy of the proposed PO compared to the other well-known competing optimizers. The convergence rates show good, fast, and stable convergence rates with lower RMSE via PO compared to the other five competitive optimizers. Moreover, the lower mean realized via the PO optimizer is illustrated by the box plot for all optimizers.Keywords
Cite This Article
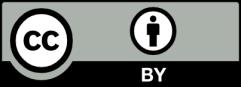
This work is licensed under a Creative Commons Attribution 4.0 International License , which permits unrestricted use, distribution, and reproduction in any medium, provided the original work is properly cited.