Open Access
ARTICLE
Steel Surface Defect Recognition in Smart Manufacturing Using Deep Ensemble Transfer Learning-Based Techniques
Department of Information and Communication Engineering, Changwon National University, Changwon, 51140, Republic of Korea
* Corresponding Author: Jongwon Seok. Email:
Computer Modeling in Engineering & Sciences 2025, 142(1), 231-250. https://doi.org/10.32604/cmes.2024.056621
Received 26 July 2024; Accepted 21 October 2024; Issue published 17 December 2024
Abstract
Smart manufacturing and Industry 4.0 are transforming traditional manufacturing processes by utilizing innovative technologies such as the artificial intelligence (AI) and internet of things (IoT) to enhance efficiency, reduce costs, and ensure product quality. In light of the recent advancement of Industry 4.0, identifying defects has become important for ensuring the quality of products during the manufacturing process. In this research, we present an ensemble methodology for accurately classifying hot rolled steel surface defects by combining the strengths of four pre-trained convolutional neural network (CNN) architectures: VGG16, VGG19, Xception, and Mobile-Net V2, compensating for their individual weaknesses. We evaluated our methodology on the Xsteel surface defect dataset (XSDD), which comprises seven different classes. The ensemble methodology integrated the predictions of individual models through two methods: model averaging and weighted averaging. Our evaluation showed that the model averaging ensemble achieved an accuracy of 98.89%, a recall of 98.92%, a precision of 99.05%, and an F1-score of 98.97%, while the weighted averaging ensemble reached an accuracy of 99.72%, a recall of 99.74%, a precision of 99.67%, and an F1-score of 99.70%. The proposed weighted averaging ensemble model outperformed the model averaging method and the individual models in detecting defects in terms of accuracy, recall, precision, and F1-score. Comparative analysis with recent studies also showed the superior performance of our methodology.Keywords
Cite This Article
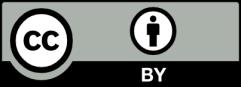
This work is licensed under a Creative Commons Attribution 4.0 International License , which permits unrestricted use, distribution, and reproduction in any medium, provided the original work is properly cited.