Open Access
REVIEW
Explainable Artificial Intelligence–A New Step towards the Trust in Medical Diagnosis with AI Frameworks: A Review
1
Department of Electronics & Telecommunication, Lavale, Symbiosis Institute of Technology, Symbiosis International
(Deemed University), Pune, 412115, India
2
Electronics & Telecommunication, Vilad Ghat, Dr. Vithalrao Vikhe Patil College of Engineering, Ahmednagar, Maharashtra,
414111, India
3
Centre for Advanced Modelling and Geospatial Information Systems (CAMGIS), School of Civil and Environmental Engineering,
Faculty of Engineering & IT, University of Technology Sydney, Sydney, 2007, Australia
4
Center of Excellence for Climate Change Research, King Abdulaziz University, Jeddah, 21589, Saudi Arabia
5
Earth Observation Centre, Universiti Kebangsaan, Institute of Climate Change, Malaysia, Selangor, 43600, Malaysia
6
Department of Computer Science, Lavale, Symbiosis Institute of Technology, Symbiosis International (Deemed University),
Pune, 412115, India
7
Symbiosis Center for Applied Artificial Intelligence (SCAAI), Lavale, Symbiosis International (Deemed University),
Pune, 412115, India
* Corresponding Author: Shilpa Gite. Email:
Computer Modeling in Engineering & Sciences 2022, 133(3), 843-872. https://doi.org/10.32604/cmes.2022.021225
Received 03 January 2022; Accepted 12 May 2022; Issue published 03 August 2022
Abstract
Machine learning (ML) has emerged as a critical enabling tool in the sciences and industry in recent years. Today’s machine learning algorithms can achieve outstanding performance on an expanding variety of complex tasks–thanks to advancements in technique, the availability of enormous databases, and improved computing power. Deep learning models are at the forefront of this advancement. However, because of their nested nonlinear structure, these strong models are termed as “black boxes,” as they provide no information about how they arrive at their conclusions. Such a lack of transparencies may be unacceptable in many applications, such as the medical domain. A lot of emphasis has recently been paid to the development of methods for visualizing, explaining, and interpreting deep learning models. The situation is substantially different in safety-critical applications. The lack of transparency of machine learning techniques may be limiting or even disqualifying issue in this case. Significantly, when single bad decisions can endanger human life and health (e.g., autonomous driving, medical domain) or result in significant monetary losses (e.g., algorithmic trading), depending on an unintelligible data-driven system may not be an option. This lack of transparency is one reason why machine learning in sectors like health is more cautious than in the consumer, e-commerce, or entertainment industries. Explainability is the term introduced in the preceding years. The AI model’s black box nature will become explainable with these frameworks. Especially in the medical domain, diagnosing a particular disease through AI techniques would be less adapted for commercial use. These models’ explainable natures will help them commercially in diagnosis decisions in the medical field. This paper explores the different frameworks for the explainability of AI models in the medical field. The available frameworks are compared with other parameters, and their suitability for medical fields is also discussed.Keywords
Cite This Article
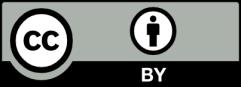
This work is licensed under a Creative Commons Attribution 4.0 International License , which permits unrestricted use, distribution, and reproduction in any medium, provided the original work is properly cited.