Open Access
ARTICLE
Frequencies Prediction of Laminated Timber Plates Using ANN Approach
1 School of Resources, Environment and Materials, Guangxi University, Nanning, 530004, China
2 Department of Civil and Environmental Engineering, University of Alberta, Edmonton, AB, T6G 1H9, Canada
* Corresponding Author: Jan Niederwestberg. Email:
Journal of Renewable Materials 2020, 8(3), 319-328. https://doi.org/10.32604/jrm.2020.08696
Received 26 September 2019; Accepted 13 January 2020; Issue published 01 March 2020
Abstract
Cross laminated timber (CLT) panels, which are used as load bearing plates and shear panels in timber structures, can serve as roofs, walls and floors. Since timber is construction material with relatively less stiffness, the design of such structures is often driven by serviceability criteria, such as deflection and vibration. Therefore, accurate vibration and elastic properties are vital for engineered CLT products. The objective of this research is to explore a method to determine the natural frequencies of orthotropic wood plates efficiently and fast. The method was developed based on vibration signal processing by wavelet to acquire the effective sample data, and a model developed by artificial neural network (ANN) to achieve the prediction of nature frequencies. First, experiments were performed to obtain vibration signals of single-layer plates. The vibration signals were then processed by wavelet packet transform to extract the eigenvectors, which served as the samples to train the ANN model. The trained model was employed to predict three nature frequencies of other test specimens. The results showed that the proposed method can produce predicted frequencies fast and effi- ciently within 10% of the measured values.Keywords
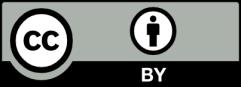