Open Access
ARTICLE
Performance Analysis of Machine Learning Algorithms for Classifying Hand Motion-Based EEG Brain Signals
1 Department of Computer Science, College of Applied Studies, King Saud University, Riyadh, 11495, Saudi Arabia
2 Department of Computer Science and Engineering, Manipal University Jaipur, Jaipur, 303007, India
3 Department of Computer Science, CHRIST (Deemed to be University), Bangalore, 560029, India
4 Department of Computer Science and Engineering, CHRIST (Deemed to be University), Bangalore, 560074, India
5 Information Systems Department, Imam Mohammad Ibn Saud Islamic University (IMSIU), Riyadh, 11432, Saudi Arabia
* Corresponding Author: Abdul Khader Jilani Saudagar. Email:
(This article belongs to the Special Issue: Healthcare Intelligence using Deep Learning and Computer Vision)
Computer Systems Science and Engineering 2022, 42(3), 1095-1107. https://doi.org/10.32604/csse.2022.023256
Received 01 September 2021; Accepted 12 October 2021; Issue published 08 February 2022
Abstract
Brain-computer interfaces (BCIs) records brain activity using electroencephalogram (EEG) headsets in the form of EEG signals; these signals can be recorded, processed and classified into different hand movements, which can be used to control other IoT devices. Classification of hand movements will be one step closer to applying these algorithms in real-life situations using EEG headsets. This paper uses different feature extraction techniques and sophisticated machine learning algorithms to classify hand movements from EEG brain signals to control prosthetic hands for amputated persons. To achieve good classification accuracy, denoising and feature extraction of EEG signals is a significant step. We saw a considerable increase in all the machine learning models when the moving average filter was applied to the raw EEG data. Feature extraction techniques like a fast fourier transform (FFT) and continuous wave transform (CWT) were used in this study; three types of features were extracted, i.e., FFT Features, CWT Coefficients and CWT scalogram images. We trained and compared different machine learning (ML) models like logistic regression, random forest, k-nearest neighbors (KNN), light gradient boosting machine (GBM) and XG boost on FFT and CWT features and deep learning (DL) models like VGG-16, DenseNet201 and ResNet50 trained on CWT scalogram images. XG Boost with FFT features gave the maximum accuracy of 88%.Keywords
Cite This Article
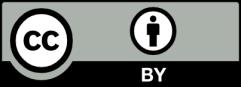
This work is licensed under a Creative Commons Attribution 4.0 International License , which permits unrestricted use, distribution, and reproduction in any medium, provided the original work is properly cited.